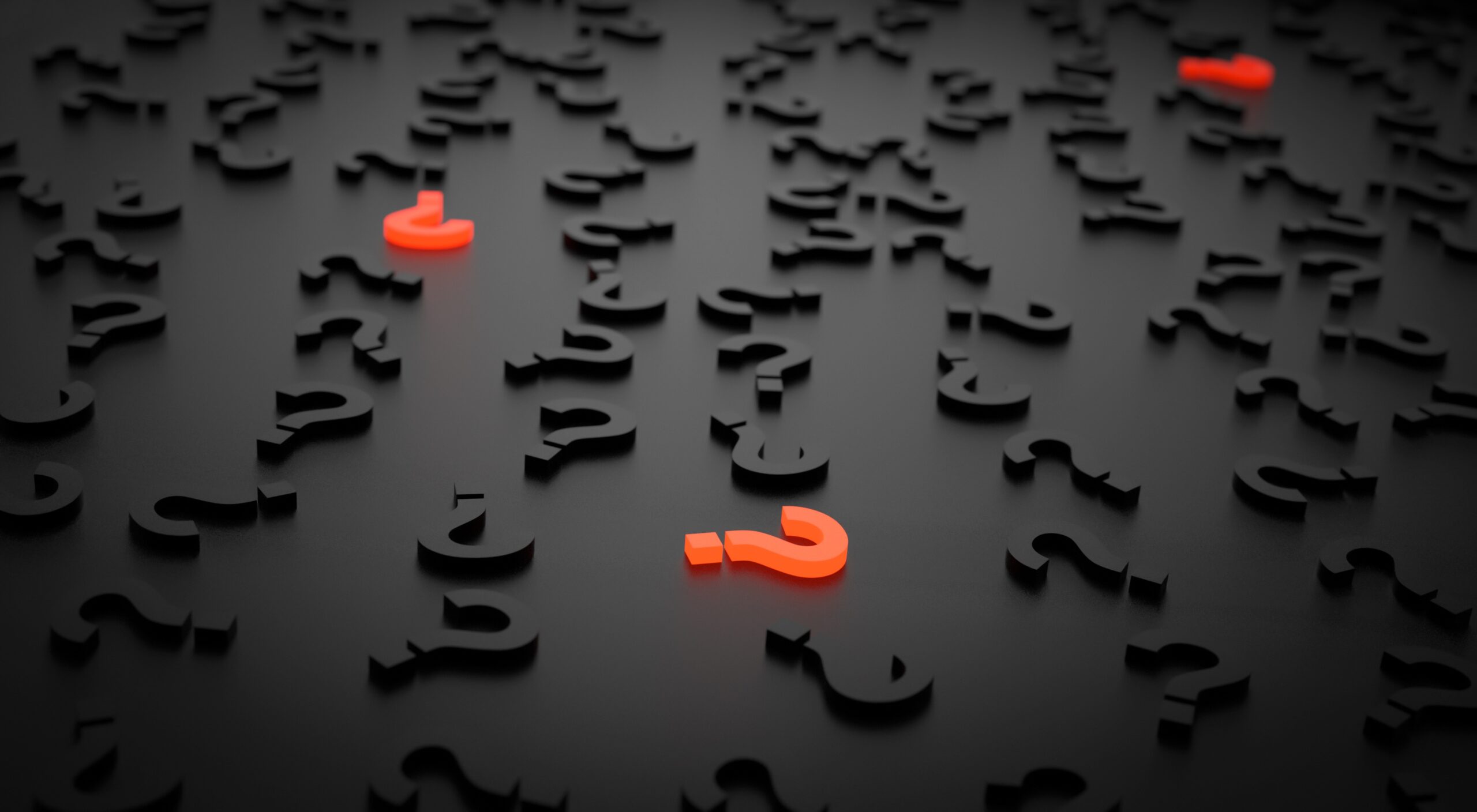
Blog Post
How to Achieve Effective Data Discovery: The Four Essential Elements
In this post we’ll explain the four essential elements of an effective data discovery program.
The most effective data discovery programs are:
- Specific — targeting well-defined data types
- Comprehensive — cover a broad scope of systems and file types
- Evidence-based — use a sensitive data discovery tool capable of producing consistent, reliable results
- Repeatable — can be performed periodically to manage and monitor sensitive data risk over time
Specific: Define Your Data Discovery Goals
The first step of data discovery is understanding the information you want to find; the “data types” you need to identify. You also need to understand the wider purpose of the data discovery program.
For example, if you are working toward PCI DSS v4.0, your discovery program will be limited to pre-paid, debit and credit card data types.
However, most organizations have obligations beyond PCI DSS including privacy compliance and cybersecurity goals that extend to personal information, company proprietary and other forms of sensitive data. This may mean you need to scan for multiple different data types. These could be personal data such as social security numbers, passport IDs, names and birth dates. It could also be non-personal information including intellectual property, company records or software code.
Good discovery tools will be capable of identifying a wide range of personally identifiable data (PII), payment card information and allow for discovery of custom data types. They will also support concurrent discovery of multiple data types during a single scan.
Comprehensive: Define the Scope of Your Discovery Scan
Once you have a clear understanding of the data types you’re looking for, you need to determine the systems and services you want to scan. This may extend to your whole network and cloud-based infrastructure or be limited to specific systems or environments.
It’s important to ensure your discovery tool supports all the components you need to scan.
Where there’s a compliance incentive driving your discovery program, the more comprehensive your scope the better. There are common places sensitive data tends to turn up in discovery scans, so you need to make sure these are also included in your scope:
- Collaboration tools such as Teams, OneNote, SharePoint
- Deleted files, including those within recycle bin, slack space and unallocated sectors
- Log files, typically in verbose logs used for troubleshooting
- Shadow files, including temporary and recovery files as well as shadow volumes
- User generated files, including compressed and archived files as well as in-use files
- Volatile memory such as cache memory and RAM
Evidence-Based: Use a Sensitive Data Discovery Tool
Some organizations rely on procedural methods such as data flow diagrams to identify data within their networks. Data flow diagrams show us the design of processes and how data is intended to move between systems, teams and third parties. However, data flow diagrams can’t identify data that has been captured and stored beyond these designed processes.
It is possible to perform basic data discovery using RegEx scripts, but these are prone to high error rates, both in failing to identify matching data types with minor pattern variations and in identifying false positives.
Sensitive data discovery tools are able to overcome these limitations through programmatic and context-based pattern matching to produce more accurate results.
It’s also important to understand how your chosen discovery tool manages data during the discovery process. While some tools take a copy of the data to scan it on third-party infrastructure, tools designed to support privacy compliance and cybersecurity will perform discovery scanning in situ, so there is no unnecessary duplication or processing of data outside your organization’s control.
Repeatable: Automate Data Discovery to Manage Data Risk
Companies ingest new information every and new threats emerge all the time. Establishing an effective data discovery program as part of a repeatable, periodic process provides a strong foundation for managing data risk.
Tools that support automation of this process are invaluable, since budget and resource constraints mean organizations are unable to sustain this oversight otherwise. Not only can automating discovery ensure you know where your data is, but it also aids rapid remediation and management of rogue data and newly identified data repositories.
Further, the standardized reporting and risk profiling offered by leading data discovery tools can be used to monitor and measure data risks on an ongoing basis, as well as providing evidence for audit purposes and legal and regulatory compliance.
To get started on your data discovery journey, book a call with one of our experts today.