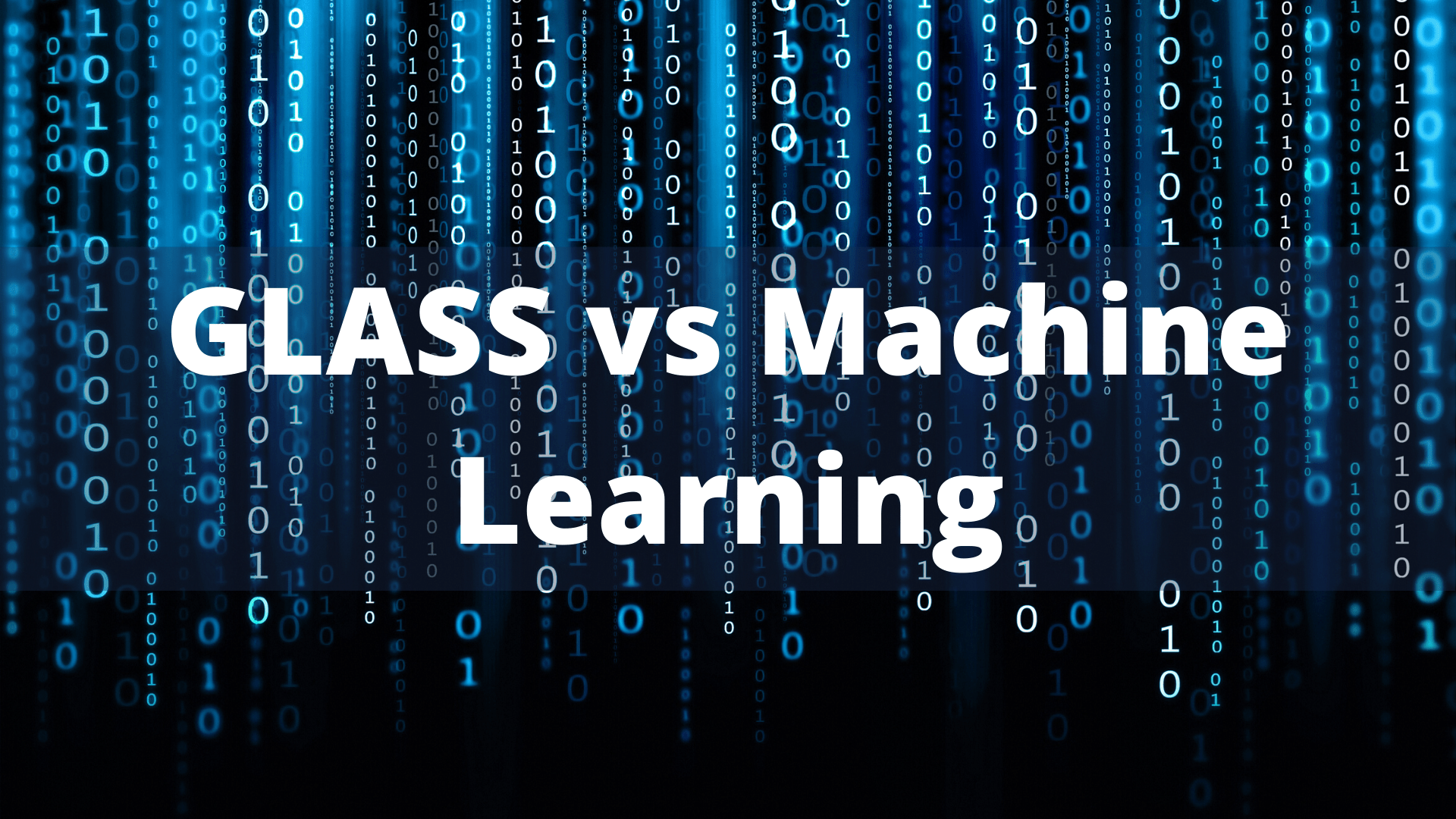
AI/ML
Take machine learning software for a test drive before buying
What is Machine Learning?
Machine learning is the latest buzzword to encompass the idea of throwing a wide variety of data at a general computer algorithm such as a neural network. In most instances, it makes a decision, and the user tells it whether it’s right or wrong. Over time, the computer algorithm learns the specific trends and is eventually able to detect right vs wrong on its own. But manually teaching a machine what is right vs wrong is a long and tedious process. Not to mention, a process that is extremely prone to error. A historic example of when machine learning goes wrong is Microsoft’s attempt to create an AI chat robot named Tay on Twitter, which quickly became an “evil” bot thanks to the vulgar information being fed to her.
The age-old term, “garbage in, garbage out” has never held more truth than it does for machine learning. If you map this concept to the detection of sensitive data across millions of files and combine it with input from hundreds or thousands of employees who may not completely understand the data they are looking at, machine learning platforms could quickly be trained to ignore genuine findings, while displaying a higher ratio of incorrect findings, resulting in a substantial amount of time wasted.
Machine learning gives vendors the ability to put together a very tenuous framework, where they shift the responsibility of solving search problems from themselves to the customer. This is seen in two examples, the first being when the algorithm detects too many false positives (and it will if the user incorrectly inputs the data). The vendor may then place blame on the customer for not having enough training. Another scenario can occur when the software doesn’t detect legitimate matches because it hasn’t been trained to accurately find this data; therefore, the vendor can still place blame on customer user error.
Ground Lab’s Answer to the Machine Learning Pitfall: GLASS™ Technology
At Ground Labs, we work diligently to avoid scenarios like these. We have designed our proprietary data discovery language, GLASS™ Technology, to identify exactly what to look for based on over a successful 10-year company track record. We are able to quickly and accurately detect what does, or does not constitute as a genuine match. In the unique situations where a false positive is found in an environment, GLASS Technology empowers our solution, Enterprise Recon, to provide a very simple workflow to flag the matches and ensure they never appear again.
To help others avoid the misconceptions and biases around machine learning-based systems, we’ve put together the following recommendations:
1 – Understand the truth of what machine learning is and identify the true shortcomings of the technology. If it sounds too good to be true, it usually is.
2 – Test a machine learning-based platform in a real environment and compare its results to Enterprise Recon.
3 – Ensure the testing covers a variety of real situations – a desktop, a server, a database, an email account, a cloud storage target.
Once you understand these tenets, you’ll be able to look beyond the marketing hype and compare GLASS™ Technology over Machine Learning and how each performs in your environment. It’s important, especially in today’s high-stakes security and compliance landscape, to have the software and partners you can trust.
Interested in learning more about how Enterprise Recon is the best data discovery solution on the market? Learn more here.